AI audio stem splitting is transforming the way producers work—just as our Ultimate Guide to Producing Rap Beats at Home shows, innovative techniques are democratizing the production process for bedroom producers and professionals alike.
There’s a revolution whispering in the back alleys of our sound systems—a distortion so subtle yet seismic it’s shaking the very foundations of music production. Picture this: a track pulsating with raw energy, every note a fierce heartbeat of a culture that’s always been about breaking boundaries. Now, imagine that same track ripped apart by algorithms, its inner workings exposed in a dazzling display of technical wizardry. Welcome to the world of AI audio stem splitting.
This isn’t your grandma’s remastering process. We’re talking about a tool that’s as much about art as it is about science—a raw, relentless force that’s challenging everything from how we produce music to how we experience it. AI audio stem splitting is the wild child of digital signal processing, a technology that dissects songs into their individual elements—vocals, bass, drums, and more—with a precision that’s both awe-inspiring and unsettling. But before we get lost in the binary labyrinth, let’s crank up the volume and break down the scene.
Breaking the Sound Barrier: A Raw Introduction
In the underbelly of modern music production, the rules are meant to be bent, if not broken entirely. This digital revolution isn’t just about clean cuts or pristine sounds; it’s about raw energy, unpredictability, and the power to reimagine the way art is created and consumed. AI audio stem splitting stands at the crossroads of technology and culture, a tool that can isolate every nuance of a track and offer producers unprecedented control over their sonic landscapes.
Think about it: for decades, producers have struggled with messy multitracks, fighting to pull the perfect vocal line or isolate that perfect bass groove. Enter AI models like Spleeter—open source and unrelenting in their precision. Suddenly, what was once a painstaking process becomes a playground for creativity. But here’s the kicker: with great power comes great controversy. Is this the democratization of music, or the death knell for traditional craftsmanship?
It’s a question that rips through the fabric of the music industry like a rebellious guitar riff cutting through a quiet, somber night. And as we stand on the brink of this AI revolution, we need to ask ourselves: who truly benefits from these innovations, and what do we lose in the process?
A Cultural Collision: The Beat, the Code, and the Streets
Let’s take a moment to drop into the cultural context that makes this issue so electrifying. In the heart of hip-hop and underground electronic scenes, every beat tells a story—a narrative of struggle, triumph, and raw emotion. Music has always been more than just sound; it’s a living, breathing entity that reflects our times. It’s been a vehicle for revolution, a cry against injustice, and a testament to the human spirit.
Now, imagine that same cultural pulse being dissected by algorithms trained on thousands of hours of music data. On one hand, you have a tool that can pull out the exact vocal line of your favorite rapper, isolate the bass that vibrates through the soul of a dancefloor, or extract the atmospheric ambiance of a reverb-soaked guitar solo. It’s like having a backstage pass to the soul of a song, exposing the anatomy of art with unflinching clarity.
But here’s where it gets messy: the same technology that empowers can also emasculate. Traditionalists and purists in the industry argue that the essence of music lies in its imperfections—its raw, unfiltered, and unpredictable nature. When you let an algorithm dissect a track, are you not, in a way, stripping it of its humanity? The organic struggle between human intuition and digital precision plays out like a dissonant chord, leaving us to wonder if this is progress or a shortcut that kills the craft.
And yet, we’re in too deep to turn back now. The beat goes on, and in the tangled web of code and culture, every split stem carries with it the weight of history, the promise of innovation, and the bittersweet taste of what might be lost.
Under the Hood: The Science of Audio Stem Splitting
Let’s get technical—really technical. Behind the sleek interfaces and impressive demos, AI audio stem splitting is driven by deep learning models that operate like intricate digital scalpel blades, slicing through layers of sound with mathematical precision. At the core of many of these models lies a structure reminiscent of the famed U-Net architecture, originally designed for image segmentation. Adapted for audio, these models have evolved to navigate the labyrinth of frequencies, amplitudes, and temporal dynamics that constitute a track.
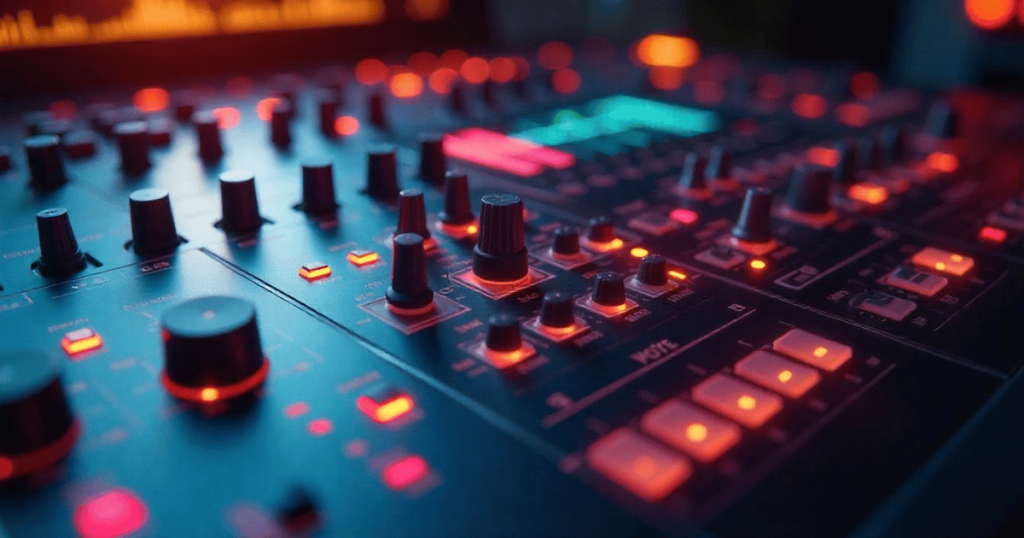
The U-Net Revolution
U-Net’s architecture is a story of contrasts—a blend of contraction and expansion, like the rise and fall of a symphonic journey. Originally built for biomedical image segmentation, it has found a new life in audio processing. The model works by compressing the input data into a lower-dimensional space, capturing essential features, and then expanding it back to its original dimensions. This process allows the model to separate the mixed signals into distinct stems.
In practical terms, you feed the model a stereo audio file, and it begins its work, analyzing the spectral components of the track. The algorithm learns to recognize patterns: the consistent pitch of a vocal line, the repetitive rhythm of a drum beat, the sustained notes of a guitar. Through layers of convolutional neural networks (CNNs) and recurrent neural networks (RNNs), it identifies and isolates these patterns, effectively “splitting” the audio into its constituent parts.
But here’s the raw truth—this isn’t magic. It’s machine learning, with all the quirks and biases that come with it. Training these models requires vast amounts of data—thousands of hours of music, meticulously labeled and processed. And even then, the results aren’t perfect. There are always artifacts, ghostly remnants of the original mix that remind you that the algorithm is, after all, a tool built by humans, inheriting all our imperfections.
Open Source Titans: Spleeter and Beyond
Spleeter, developed by Deezer, is arguably the poster child of open source AI audio stem splitting. Released to the public with a promise of accessibility and transparency, it quickly became a favorite among producers, remixers, and music enthusiasts alike. Spleeter uses pre-trained deep neural networks to separate audio files into two, four, or five stems, catering to various levels of complexity and user needs.
Its impact is undeniable. Suddenly, independent artists and bedroom producers gained access to a tool that was once the preserve of high-budget studios. The democratization of audio processing sparked a renaissance in remix culture and opened up avenues for creative experimentation that were previously unimaginable. But as with every revolution, there’s a flip side: while accessibility soared, the boundaries of originality and craftsmanship became blurred.
Other models and tools have since emerged, each pushing the envelope further. Projects like Open-Unmix and Demucs have taken up the mantle, incorporating advancements in signal processing and neural network architectures to refine the separation process. They bring their own flavor to the table, challenging Spleeter’s dominance and adding layers of complexity to the debate on AI’s role in music production.
Data Science Deep Dive
Let’s peel back another layer and talk data science. The process of audio stem splitting isn’t just about dividing sounds—it’s a data-intensive operation that leverages advanced statistical techniques and signal processing algorithms. At its heart is the Fourier Transform, a mathematical tool that converts time-domain signals into frequency-domain representations. This transformation is the bedrock upon which neural networks operate in audio analysis.
Once in the frequency domain, the model employs spectrogram analysis, breaking the sound into a matrix of frequency versus time. It’s here that the neural networks flex their muscles, scanning for patterns that correspond to different instruments or vocal lines. The model is trained on loss functions specifically designed to minimize the interference between separated stems. In simpler terms, it’s a battle against noise and overlap, a relentless pursuit of clarity.
The training phase is where data science meets the art of trial and error. Researchers meticulously curate datasets, often annotating tracks by hand to ensure the model learns the subtle differences between overlapping sounds. This process is both a technical challenge and an artistic endeavor, as the data itself is imbued with the soul of the music it represents.
Even with all this sophistication, the models are far from perfect. They often struggle with genres that defy conventional structures, where improvisation and organic interplay reign supreme. Jazz, experimental electronic, and live recordings are notorious for throwing the algorithms off balance. These imperfections are a stark reminder that while AI can dissect and analyze, it can’t fully grasp the emotional and cultural resonance of music.
The Real World Impact: From Studio to Street
AI audio stem splitting isn’t confined to academic papers or Silicon Valley labs—it’s making waves in the real world, reshaping industries and challenging established norms. Let’s dive into some real-world examples that illustrate both the promise and the peril of this technology.
Remix Culture and the Rise of the Bedroom Producer
In the early days of digital music production, remixing was a niche art form, reserved for those with access to expensive equipment and exclusive studio sessions. Fast forward to today, and AI-powered tools have leveled the playing field. The bedroom producer, armed with nothing more than a laptop and a deep passion for music, can now isolate, manipulate, and reassemble tracks with surgical precision.
Take the case of underground hip-hop artists who sample obscure records, flipping them into entirely new creations. AI audio stem splitting has become the secret sauce behind countless remixes that circulate online, transforming forgotten beats into viral sensations. But this democratization comes with a catch. As the line between homage and plagiarism blurs, debates rage about the ethics of sampling and the erosion of traditional production techniques.
Live Performances and Interactive Experiences
Beyond the realm of recording studios, live performances are also feeling the tremors of AI innovation. Imagine a DJ set where, in real time, the music is deconstructed and reassembled based on the crowd’s energy. AI can analyze the beat, isolate vocals, and even suggest on-the-fly modifications to keep the dancefloor pulsating. It’s a wild, experimental frontier that marries technology with the raw, unpredictable energy of live performance.
Festivals and clubs have become testing grounds for these innovations, where technology isn’t just a tool—it’s an integral part of the show. The audience is no longer a passive recipient but an active participant in a dynamic, ever-evolving musical narrative. Yet, this spectacle of technology raises fundamental questions: When the magic of live music is mediated by algorithms, what happens to the spontaneous connection between artist and audience?
Commercial Applications and Industry Disruption
It’s not just the indie scene that’s being upended. Major labels and streaming platforms are diving headfirst into AI, using audio stem splitting to enhance everything from music recommendation engines to automated remix contests. The ability to isolate and analyze individual components of a track allows for more nuanced metadata tagging, improved sound quality enhancements, and even personalized listening experiences.
However, as with any disruptive technology, there’s a darker side. The rise of AI-driven tools has sparked fears of job displacement among traditional audio engineers and producers. What happens to the human touch in a craft that has always been about intuition, experience, and a deep emotional connection to the music? While the promise of efficiency and innovation is tantalizing, the human cost cannot be ignored.
The Double-Edged Sword: Progress or Shortcut?
Every technological breakthrough carries with it an inherent contradiction—a tool for progress that might also serve as a shortcut, a facilitator that simultaneously undermines the craft it aims to support. AI audio stem splitting is no exception. On one hand, it’s an enabler, liberating creativity from the constraints of time-consuming manual editing. On the other, it’s a potential eraser of nuance, a force that risks diluting the very essence of artistic expression.
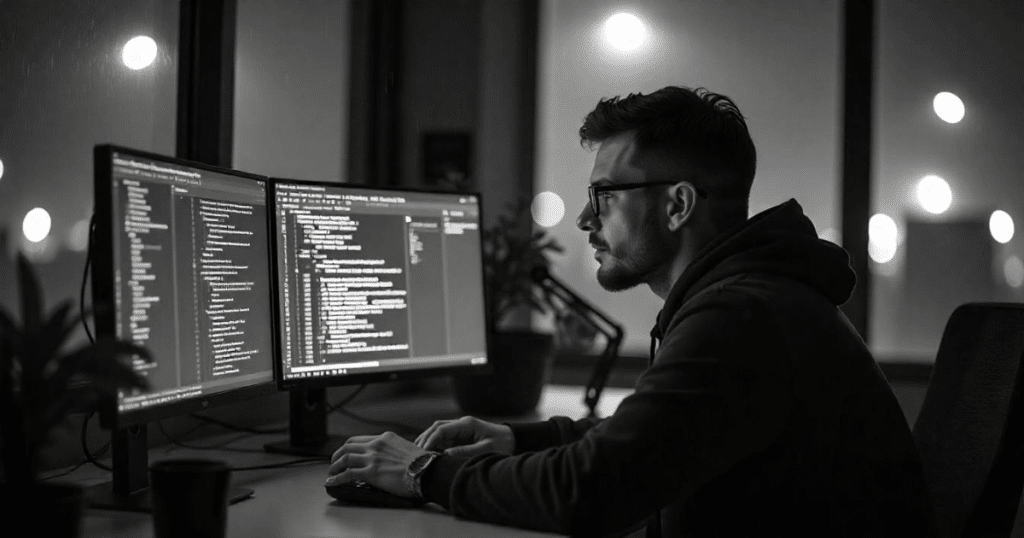
The Empowerment Narrative
For many, AI audio stem splitting represents a triumph of human ingenuity—a way to harness the power of advanced algorithms to unlock new creative possibilities. Independent artists, once shackled by the limitations of their hardware and budgets, are now able to explore soundscapes that were once the exclusive domain of well-funded studios. It’s a democratization of artistry that celebrates the convergence of technology and creativity.
The empowerment narrative is strong. It’s a rallying cry for innovation, a call to break free from the old guard and embrace the future. In a world where traditional barriers are crumbling, AI offers a promise of unlimited potential, a chance for anyone with a vision to create something extraordinary.
The Shortcut Conundrum
Yet, as we celebrate this newfound freedom, we must also confront the possibility that these tools might be turning into crutches—a shortcut that undermines the rigorous craft of music production. When an algorithm can isolate a vocal track with the click of a button, does it not risk stripping away the sweat, the trial and error, the serendipitous accidents that often give music its soul?
There’s a raw, unpolished truth to consider: art isn’t just about precision; it’s about imperfection, the unpredictability of human creativity. The very imperfections that AI seeks to eliminate are what give music its character. In the pursuit of clarity, we might inadvertently wash away the grit and soul that make a track truly resonate.
And then there’s the ethical dilemma. With AI tools becoming ubiquitous, what happens to originality? When every producer has access to the same high-powered tool, the lines between inspiration, imitation, and outright appropriation blur. It’s a debate that cuts to the core of what it means to be an artist in the digital age—a collision between the relentless march of progress and the timeless, often messy, human experience of creation.
In the Trenches: Technical Data Science and Open Source Frontiers
Let’s get even deeper into the labyrinth of data science, where the code meets the culture. For those who’ve ever peeked under the hood of an AI model, the world of audio stem splitting is a playground of matrices, loss functions, and neural network layers that often defy intuitive understanding.
Architecture and Algorithms
At its core, the process begins with the transformation of an audio signal from its raw time-domain form into a spectral representation via the Short-Time Fourier Transform (STFT). This step is critical—it breaks the sound into its frequency components, enabling the model to analyze the nuances of each instrument or vocal line. Imagine the STFT as the prism that splits white light into a rainbow, revealing the hidden colors within a seemingly uniform beam.
Once in the frequency domain, the neural network takes over. Convolutional layers scan through the spectrogram, learning to identify recurring patterns—those distinct harmonic signatures that differentiate a drum beat from a bass line. The U-Net architecture, with its encoder-decoder structure, then steps in, condensing and reconstructing the data in a way that isolates each component. This is where the model’s training comes into play, refined through countless iterations on diverse datasets. The impact of AI isn’t limited to just stem splitting. It’s reshaping entire production workflows—as discussed in our article on How AI and Royalty-Free Instrumentals Are Shaping Rap’s Future, advanced algorithms are now integral to modern beat-making.
The loss functions used in this training process are designed to minimize the interference between the separated stems. In other words, the model isn’t just trying to split the sound—it’s trying to ensure that each isolated track is as clean and distinct as possible. This is a delicate balancing act, one that requires a deep understanding of both the art of music and the science of sound.
1. Transforming Raw Audio into Usable Data
A. Signal Representation: Time-Domain vs. Frequency-Domain
Every audio file starts as a time-series signal—amplitudes sampled at regular intervals. However, to meaningfully separate overlapping sounds, we first convert this time-domain signal into a frequency representation using the Short-Time Fourier Transform (STFT).
STFT Formula:
The STFT of a signal x(t) is defined as:
X(τ, ω) = ∫−∞∞ x(t) · w(t − τ) · e−jωt dt
Here, w(t − τ) is a window function (commonly Hamming or Hann) centered at time τ. The transform yields a complex-valued matrix—a spectrogram—where each element encodes amplitude and phase for a specific time-frequency bin.
Magnitude and Phase:
Most algorithms focus on the magnitude spectrum, |X(τ, ω)|, which is easier to work with for separation tasks, while phase is handled separately or approximated in the inverse transform.
B. Spectrogram Analysis
The spectrogram serves as the input “image” for deep neural networks. Each pixel represents the intensity of a particular frequency at a specific time. This conversion allows us to leverage techniques from computer vision, particularly convolutional neural networks (CNNs), to identify patterns corresponding to different instruments or vocals.
2. Core Models and Architectures
A. U-Net for Audio Source Separation
The U-Net architecture is a favorite for tasks that require precise localization—in this case, isolating overlapping sound components. Originally designed for biomedical image segmentation, U-Net adapts well to spectrogram inputs.
Encoder-Decoder Structure:
The model compresses the input spectrogram into a latent space (encoder) and then reconstructs the target output (decoder). Skip connections between corresponding layers in the encoder and decoder ensure high-frequency details aren’t lost.
Mathematical Underpinning:
Consider an encoder layer that performs convolution followed by an activation function f. Given input I, the output O is:
O = f(W * I + b)
where * denotes convolution, W is the weight matrix, and b is a bias term. The decoder mirrors this process using transposed convolutions to upscale the latent representation.
B. Other Architectures: Open-Unmix and Demucs
Open-Unmix: Uses a bidirectional Long Short-Term Memory (LSTM) network to capture temporal dependencies in music, alongside feed-forward layers that operate on frequency bins extracted from the spectrogram.
Demucs: Incorporates both convolutional encoders/decoders and recurrent layers to capture long-range dependencies. It even uses time-domain processing to overcome phase reconstruction challenges that plague purely spectrogram-based methods.
3. Training Data: The Backbone of Model Performance
A. Data Collection and Annotation
Dataset Characteristics:
High-quality training datasets consist of thousands of tracks with isolated stems (vocals, drums, bass, etc.). These tracks are typically recorded in controlled studio environments where each instrument is recorded separately.
Synthetic Mixing:
In scenarios where isolated stems are not available, clean tracks can be artificially mixed to create training pairs. For instance, the MUSDB18 dataset is commonly used—it provides professionally separated stems for around 150 tracks.
B. Preprocessing Steps
Normalization: Audio signals are normalized to ensure consistency across training samples.
Windowing: The signal is divided into overlapping windows using the chosen STFT window size (e.g., 1024 samples with a 50% overlap) to create manageable spectrogram chunks.
Data Augmentation: Techniques like pitch shifting, time stretching, and adding noise are used to enrich the training set and make models robust to real-world conditions.
4. Loss Functions and Optimization
A. Objective Functions
The goal is to minimize the difference between the predicted stem and the ground truth isolated stem. Common loss functions include:
Mean Squared Error (MSE):
MSE = (1/N) ∑i=1N (Ŝi − Si)²
where Ŝi is the predicted stem value and Si is the ground truth.
L1 Loss (Mean Absolute Error):
L1 Loss = (1/N) ∑i=1N |Ŝi − Si|
This is sometimes preferred because it’s less sensitive to outliers.
B. Perceptual and Adversarial Losses
Perceptual Loss: In some cases, losses computed on features extracted from pre-trained audio recognition networks (akin to perceptual losses in computer vision) are added to better capture human audio perception nuances.
Adversarial Training: A GAN-like approach can be used where a discriminator is trained to distinguish between real and generated stems, pushing the generator (separation network) to produce more realistic outputs.
5. Feature Engineering and Model Inputs
A. Input Features
Magnitude Spectrogram: Primary input, sometimes augmented with additional features like Mel-frequency spectrograms or chromagrams which capture musical timbre and harmony.
Phase Information: Although less commonly used, some approaches integrate phase or use phase reconstruction algorithms (e.g., Griffin-Lim) during the inverse transformation.
B. Temporal Context
Context Windows: Models often process multiple consecutive frames to capture context. This is critical for distinguishing between transient drum hits and sustained vocals.
Recurrent Layers: LSTM or GRU layers capture dependencies across time, enabling the model to understand the evolution of sound across frames.
6. Training Process and Algorithmic Considerations
A. Batch Processing and Data Shuffling
Mini-Batch Training: Audio segments (typically represented as spectrogram patches) are processed in batches to efficiently compute gradients via backpropagation.
Shuffling and Randomization: Critical for avoiding overfitting to specific musical passages or genres. Data shuffling ensures that each mini-batch is representative of the overall diversity in the dataset.
B. Optimization Algorithms
Adam Optimizer: Widely used due to its adaptive learning rate capabilities. Its update rule combines the benefits of AdaGrad and RMSProp:
θt+1 = θt − (η / (√(v̂t) + ε)) · m̂t
where m̂t and v̂t are bias-corrected first and second moment estimates, respectively.
Learning Rate Schedules: Learning rates are often decayed over time or adjusted via scheduling algorithms to ensure convergence during long training periods.
C. Evaluation Metrics
Signal-to-Distortion Ratio (SDR): A key metric to assess the quality of source separation:
SDR = 10 · log10 ( ||starget||² / ||enoise||² )
where starget is the true signal and enoise is the error introduced by the separation.
Signal-to-Interference Ratio (SIR) and Signal-to-Artifacts Ratio (SAR): These metrics further dissect the types of errors present in the separated outputs, providing a granular view of model performance.
7. Real-World Examples and Experimentation
A. Case Study: Spleeter
Architecture: Spleeter utilizes a simple yet effective U-Net variant, with convolutional layers downsampling the spectrogram followed by a mirrored upsampling path. Each convolutional layer is paired with batch normalization and ReLU activations to ensure stable training.
Training Data: It was trained on datasets like MUSDB18, which provided isolated stems for each track. Data augmentation techniques were applied to broaden its generalizability.
Output Quality: While Spleeter is effective on many genres, its performance can degrade on complex mixes with heavy reverb or overlapping instruments, highlighting the ongoing challenge of capturing all the nuances in diverse audio sources.
B. Experimenting with Custom Architectures
Researchers often experiment with hybrid models that combine both time-domain and frequency-domain processing. For instance:
Hybrid Demucs: A model that processes raw audio directly in the time domain alongside spectrogram-based branches. This dual approach leverages the benefits of both representations, often using loss functions computed in both domains to ensure consistency.
Algorithm Tinkering: Experimentation involves modifying kernel sizes, the number of layers, and even integrating attention mechanisms to better capture dependencies across the spectrogram. Techniques like residual connections and dropout are standard fare to combat overfitting and improve generalization.
8. Challenges and Future Directions
A. Phase Reconstruction
The Phase Problem: Many current models focus on the magnitude spectrum, leaving phase as an afterthought. Accurate phase reconstruction is crucial for high-fidelity audio but remains a challenging inverse problem.
Griffin-Lim Algorithm: A popular iterative method for phase recovery. However, it’s computationally intensive and may not always yield perfect results. Research continues into integrating phase estimation directly into end-to-end networks.
B. Handling Diverse Genres
Genre-Specific Models: Music genres vary widely in their spectral and temporal characteristics. Training a universal model is challenging; hence, domain-specific fine-tuning is often necessary.
Transfer Learning: Leveraging pre-trained models on large datasets and fine-tuning on niche genres or even individual artists’ works is an emerging trend. This helps in adapting to specific timbres and stylistic nuances.
C. Computational Complexity
Resource Constraints: Training these models demands significant computational power—GPUs or even TPUs—to process the voluminous data and complex architectures. Model compression techniques, such as quantization and pruning, are under active research to enable real-time applications on consumer hardware.
Open Source as a Catalyst for Innovation
The open source movement has been a driving force behind many of these advancements. Projects like Spleeter, Open-Unmix, and Demucs aren’t just technological tools—they’re communal endeavors, built on collaboration, transparency, and the shared desire to push the boundaries of what’s possible. Open source allows for rapid iteration and community-driven improvements, creating a feedback loop that accelerates innovation in ways that proprietary models simply cannot match. The democratization of music production is a double-edged sword. For more on navigating this evolving landscape, check out our insights in Best AI Tools for Rappers & Producers 2025, where we explore the benefits and challenges of AI-driven production.
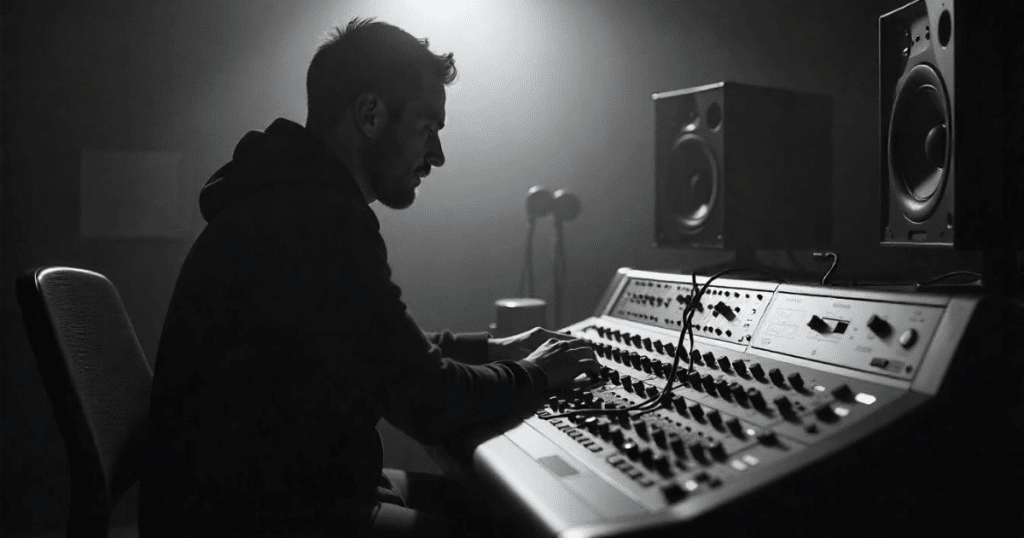
These projects serve as laboratories for experimentation, where ideas are tested, refined, and sometimes discarded in the pursuit of perfection. They’re the digital equivalent of a grassroots movement, where every coder, producer, and curious mind can contribute to a collective vision of a future where technology and art coexist in a dynamic, often unpredictable dance.
The Data Behind the Beats
Digging even deeper, we find that the backbone of these models is data—vast, unending streams of audio files, meticulously annotated and fed into the hungry algorithms. Each track, with its unique blend of instruments, vocals, and ambient noise, becomes a data point in a colossal matrix that the model learns to navigate. The richness of the data determines the model’s ability to generalize, to handle the messy, unpredictable world of real-world audio.
It’s a process fraught with challenges. Noise, overlapping frequencies, and the inherent imperfections of analog recordings all serve as obstacles that the model must overcome. The result is a tool that is as much a reflection of the data it’s been trained on as it is a product of human ingenuity. And herein lies the beauty—and the danger—of AI audio stem splitting. It mirrors the world of music in all its glorious complexity, with all the strengths, weaknesses, and contradictions that come with it.
Cultural Echoes and the Evolution of Sound
As we step back from the technical minutiae, it’s impossible to ignore the broader cultural reverberations of this technology. AI audio stem splitting isn’t just a tool for remixers or engineers—it’s a cultural catalyst, a force that’s reshaping how we understand and interact with music.
A Nod to the Legends
The history of music is studded with pioneers who redefined the soundscape of their eras. Think of the trailblazers of hip-hop, whose innovative sampling techniques turned dusty vinyl into chart-topping hits, or the experimental electronic artists who pushed the boundaries of what could be considered music. AI audio stem splitting pays homage to these legacies even as it forges its own path. It’s a continuation of a tradition that celebrates both innovation and the unpredictable, raw energy of creation.
There’s a palpable tension in the air—a collision between the reverence for analog warmth and the allure of digital precision. It’s a conversation that’s as old as the mediums themselves. And as AI tools become more sophisticated, the debate only intensifies: Can technology truly capture the soul of a performance, or does it risk reducing a vibrant, living art form to a series of data points?
The Beat Goes On: Real-World Reflections
In the gritty studios of urban centers and the cozy corners of independent record shops, the impact of AI is already being felt. Producers are experimenting with hybrid workflows that blend traditional techniques with digital wizardry. A sample might be drawn from an old jazz record, isolated with surgical precision by an AI model, and then recontextualized in a modern trap beat that vibrates with the energy of the streets. It’s a melding of eras—a dialogue between the past and the present, where each beat carries echoes of history and whispers of the future.
At the same time, there’s a palpable sense of unease. Purists argue that the magic of music lies in its imperfections—the analog crackle, the subtle fluctuations that can’t be replicated by an algorithm. For them, the reliance on AI tools represents a shift towards a sanitized, overly engineered sound that risks erasing the very human elements that make music so captivating. It’s a raw, unfiltered debate—a clash of ideologies where progress and preservation collide in a cacophony of opinions.
Confronting the Contradictions: Who Really Wins?
Let’s not sugarcoat it—AI audio stem splitting is a double-edged sword, and its impacts are as unpredictable as a freestyle rap session on a smoky backstreet. The technology opens up exhilarating possibilities while simultaneously igniting fears of cultural erasure and creative homogenization.
The Creators Versus the Gatekeepers
On one side of the ring, you have the creators: the bedroom producers, the indie remixers, the underground visionaries who see AI as a tool of liberation. For them, these technologies are not just technical marvels; they’re instruments of empowerment, democratizing a process that was once reserved for those with deep pockets and exclusive connections.
Flip the coin, and you have the gatekeepers—established artists, veteran producers, and industry insiders who view AI audio stem splitting as a shortcut that undermines the painstaking craft of music production. To them, the tool is a symptom of a larger trend: the relentless pursuit of efficiency at the expense of artistic integrity. It’s a raw, unvarnished clash of philosophies that cuts to the heart of what music is supposed to be—a testament to human creativity, with all its messy, unpredictable beauty.
The Economic Equation
Beyond the cultural and artistic debates lies a more pragmatic concern: economics. The music industry is a beast fueled by trends, technology, and a constant race for the next big hit. AI audio stem splitting has the potential to upend traditional revenue streams, from production to live performance. With the ability to manipulate tracks at will, the power dynamics shift. Who benefits when an algorithm can produce studio-quality separations in seconds? Major labels, streaming platforms, and tech companies might reap the rewards, while the nuanced artistry of traditional production could be relegated to a nostalgic footnote.
There’s a bitter irony in it all. The very tools that promise to democratize creativity might also be the harbingers of a homogenized, overly optimized sound—a future where every track is engineered for perfection, yet somehow devoid of the raw, human imperfections that once made it iconic.
Ethical Implications and the Road Ahead
The ethical questions are as deep as the bass lines these models isolate. Ownership, consent, and creative integrity are all up for debate. When an AI tool can extract a vocal performance from a copyrighted track, where does that leave the artist? What are the implications for intellectual property in an age where digital manipulation blurs the lines between inspiration and appropriation?
These questions don’t have easy answers. They’re part of a broader dialogue about the role of technology in our cultural lives—a conversation that touches on everything from the future of art to the nature of human expression. As AI continues to evolve, we’re forced to confront uncomfortable truths about who we are, what we value, and where we’re headed.
Unpredictability in the Age of Algorithms
There’s an inherent unpredictability to both art and technology, a chaotic beauty that defies neat categorizations. AI audio stem splitting is no exception—it embodies the restless spirit of innovation and the wild, unpredictable pulse of cultural evolution.
The Unexpected Outcomes
In many ways, the beauty of this technology lies in its capacity for the unexpected. Sure, the models are designed to isolate and refine, but in practice, they sometimes produce results that are downright surreal. Imagine a vocal track with a whisper of the original background noise, or a drum beat that carries echoes of an entirely different rhythm. These “artifacts” are not just mistakes—they’re windows into the hidden dimensions of sound, reminders that even in the digital age, there’s room for serendipity.
For producers and enthusiasts, these surprises can be both a curse and a blessing. They’re a reminder that even the most sophisticated algorithms have their limits, that there’s still an element of magic that can’t be replicated by lines of code. And in that gap between expectation and reality lies a space for experimentation, for a reimagining of what music can be.
The Sound of Resistance
This isn’t just a technical marvel—it’s a cultural battleground. The unpredictable nature of AI audio stem splitting reflects a deeper resistance against a sanitized, overly controlled music industry. It’s a rebellious call to arms, a reminder that the spirit of music is inherently chaotic, messy, and beautifully unpredictable. In the face of an increasingly algorithm-driven world, there’s a raw, unfiltered determination among artists to reclaim their craft, to ensure that technology serves as a tool rather than a replacement for genuine creativity.
Threads of Resistance and the Future of Music
So where does this leave us, in a world where every note can be dissected and every beat deconstructed? The future of AI audio stem splitting is as much a cultural question as it is a technical one—a tangled web of promise, peril, and endless possibility.
The Hybrid Future
Perhaps the answer lies in embracing the hybrid model—a future where human ingenuity and machine precision coalesce rather than collide. In this vision, AI becomes an extension of the artist’s toolbox, a partner in the creative process rather than an omnipotent arbiter. It’s a delicate dance, one where the imperfections of human creativity are enhanced rather than erased by the clarity of digital precision.
We’re already seeing the seeds of this future sprouting in the labs and studios of innovative creators. Artists are experimenting with workflows that blend traditional recording techniques with AI-powered enhancements, creating tracks that are both meticulously engineered and vibrantly alive. It’s a space where technology amplifies the human touch, rather than replacing it—a renegade symbiosis that holds the promise of a richer, more diverse sonic landscape.
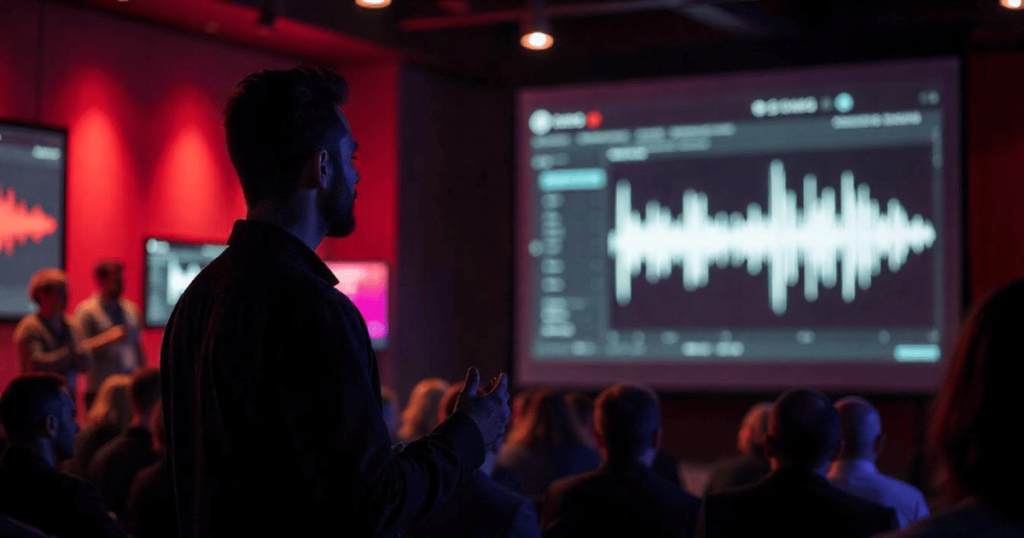
Embracing Uncertainty
There’s no neat conclusion to this story—only a series of unresolved questions, each echoing the complexity of the music itself. What happens when the tools we create begin to shape our art in ways we never anticipated? Can we preserve the raw, unpredictable spirit of creativity in an era dominated by algorithms? And as AI continues to evolve, how do we ensure that it remains a servant to human expression, rather than a master?
These are not questions with simple answers. They’re debates that will rage on in smoky studios, on crowded stages, and in the digital forums of a generation determined to carve out its own space in the annals of music history. The only certainty is that the conversation is far from over—and that every split stem, every isolated note, carries with it the weight of a cultural revolution in motion.
The Unpolished Truth: Reflections from the Front Lines
I’ve spent countless nights deep in conversation with producers, engineers, and artists who are living this revolution firsthand. Their stories are as diverse as the music they create—each one a raw, unfiltered account of what it means to navigate a landscape where technology and tradition are in constant tension.
One underground producer told me, eyes gleaming with a mix of excitement and apprehension, “It’s like getting access to a secret vault of sound. You can pull out a vocal, twist it, and turn it into something that speaks to the soul of the streets. But sometimes, I wonder if I’m losing a piece of what made it real in the first place.” That’s the heartbeat of this debate—a relentless push and pull between innovation and authenticity, between the promise of efficiency and the price of soul. Whether you’re a seasoned producer or just getting started, discover a wealth of resources in our Rap Beats and Freestyle Rap Beats sections—each packed with tips, tutorials, and industry insights to elevate your sound.
Another veteran engineer, whose career spans the analog days to the digital dawn, summed it up bluntly: “We’re standing at a crossroads. On one side, you have the ease and power of AI. On the other, the messy, unpredictable art of manual craft. And no matter how advanced the technology gets, there’s always going to be a place for that human touch.” His words resonate like a bassline, steady and unyielding, a reminder that no matter how far we dive into the digital abyss, our roots remain firmly planted in the raw, untamed soil of human experience.
Revisiting the Cultural Context: A Dialogue with History
Let’s pivot back to the cultural stage, where the echoes of the past meet the innovations of the future. Music has always been a mirror of society—a reflection of our hopes, our struggles, and our relentless desire to push beyond the boundaries of convention. In every sampled beat, every chopped and screwed vocal line, there’s a story that transcends time.
From the gritty streets of early hip-hop to the sprawling electronic soundscapes of the modern club scene, every note carries with it the weight of history. AI audio stem splitting isn’t an aberration—it’s a continuation of that tradition. It’s a tool that, for all its technical prowess, remains deeply intertwined with the cultural narratives it seeks to transform.
Imagine a scenario where a legendary vocal performance, recorded decades ago on analog tape, is resurrected through the precision of AI. The raw emotion, the subtle imperfections, the lived-in quality of that voice—all preserved and enhanced by a tool that can isolate and amplify every nuance. It’s both a tribute and a rebirth, a dialogue between the past and the present that challenges us to rethink what it means to preserve cultural heritage in the digital age.
Yet, there’s an irony here that can’t be ignored. As much as AI promises to liberate and democratize, it also threatens to commodify and dilute. The very act of dissecting a track into its bare bones raises questions about the integrity of the original art. When every element of a song can be isolated, reassembled, and repurposed at will, where does the authenticity lie? It’s a question that reverberates through every corner of the industry, a discordant note in a symphony of progress.
The Uncharted Territories: What Lies Ahead
We’re on the brink of an era defined by its unpredictability. The rapid evolution of AI audio stem splitting is only the beginning—a harbinger of deeper, more profound shifts in how we create, consume, and understand music. And as we hurtle into this uncertain future, the only constant is the unyielding pulse of innovation.
The Next Frontier of AI in Music
Beyond stem splitting, the future holds an array of possibilities that blend the digital with the analog in ways that challenge our very notion of music. Imagine AI not just as a tool for dissection, but as a creative partner—an entity that collaborates with artists to generate entirely new soundscapes, suggest chord progressions, or even predict the next big trend in music. These aren’t far-off fantasies; they’re on the horizon, shaped by the same data-driven techniques that underpin today’s stem splitting models.
The convergence of AI with other emerging technologies—virtual reality, augmented reality, and immersive audio—promises to create experiences that transcend the boundaries of traditional performance. We could soon find ourselves in concerts where the music adapts in real time to the emotions of the crowd, or interactive installations where every note is a thread in an ever-changing tapestry of sound. In these uncharted territories, the role of the artist evolves from that of a sole creator to a conductor of a digital orchestra, where technology amplifies the human spirit rather than replacing it.
Navigating the Ethical Labyrinth
With every technological leap, ethical dilemmas lurk in the shadows. The rapid advancement of AI in music challenges us to reconsider the boundaries of authorship, creativity, and ownership. When an algorithm can deconstruct and reconstruct a track with startling accuracy, the lines between original creation and derivative work blur dangerously. This isn’t just an academic debate—it has real-world implications for copyright law, revenue sharing, and the very nature of artistic expression.
We must ask ourselves: In a world where every element of a song is dissectible and repurposable, how do we preserve the rights and legacy of the original creators? The answers aren’t simple, and they require a collective reckoning with a future where technology and art are inextricably intertwined. It’s a challenge that demands not just technical innovation, but a reimagining of the cultural and legal frameworks that have long governed the music industry.
The Spirit of Rebellion
At its core, the debate over AI audio stem splitting is a rebellion—a fierce, unyielding stance against the forces of homogenization and control. It’s a call to arms for those who believe that art should remain messy, unpredictable, and profoundly human. In a digital age that increasingly prizes efficiency and uniformity, the raw, unpolished edges of creativity are more vital than ever.
This spirit of rebellion isn’t new. It’s the same fire that fueled the punk rock movement, the ethos behind underground hip-hop, and the defiant energy of countless cultural revolutions throughout history. It’s a reminder that progress, no matter how advanced, must always be tempered by a commitment to preserving the soul of the art form.
A Fragmented Future: Embracing Complexity and Uncertainty
In the end, AI audio stem splitting is both a mirror and a catalyst—a reflection of our current cultural moment and a force that will shape the future of music in ways we can scarcely imagine. It challenges the boundaries of what’s possible, forcing us to confront the contradictions inherent in every leap forward. It’s a tool that empowers and disrupts, liberates and constrains, all at once.
As we stand at this crossroads, one thing is clear: there are no easy answers, no tidy resolutions. The future of music is a tapestry of conflicting narratives, each as vital and as messy as the last. It’s a future where the old guard and the new wave collide in a cacophony of sound, where every isolated stem carries the weight of history, and where every beat is a declaration of rebellion.
The conversation is far from over. It’s a dialogue that will continue to evolve, driven by the inexorable march of technology and the indomitable spirit of human creativity. And in that ongoing exchange, there’s a raw, unfiltered energy—a promise that, no matter how advanced our tools become, the heart of music will always beat to the rhythm of the human soul.
The Beat That Won’t Die
In the dim light of a studio, under the hum of computers and the scratch of vinyl, there’s a silent revolution taking shape. AI audio stem splitting is not just a technical marvel—it’s a cultural phenomenon, a testament to our relentless pursuit of innovation and our unyielding desire to push the boundaries of what art can be.
It’s messy, it’s unpredictable, and it’s unapologetically raw. It challenges the status quo, exposes the contradictions of modern music production, and invites us all to rethink the relationship between technology and creativity. Whether you see it as a beacon of progress or a harbinger of homogenization, one thing is certain: the beat goes on, louder and more defiant than ever.
So here we are, on the brink of a sonic revolution—a wild, exhilarating journey where every isolated stem is a fragment of a larger story, a story that is still being written. And as the notes collide, diverge, and merge in a chaotic symphony of sound, we’re left with one undeniable truth: in the world of AI audio stem splitting, nothing is ever truly lost. Instead, it’s all transformed, remixed, and reborn in a relentless cycle of creation and reinvention.
A Call to Embrace the Unruly Future
To every artist, producer, and lover of music out there, consider this both a manifesto and a challenge. Embrace the raw, unpolished nature of this technology. Use it as a tool to explore new frontiers, to disrupt the predictable, and to push the boundaries of what’s possible. But never forget where you came from—the grit, the struggle, the unpredictability that makes music a living, breathing entity.
In a world increasingly dominated by algorithms and automation, it’s the human spirit, with all its messy contradictions and fierce passions, that will continue to define the soundtrack of our lives. Let AI audio stem splitting be the spark that ignites a new era of creative exploration—a call to action for those who believe that true art can never be fully captured by lines of code.
As we navigate this brave new world, let’s remain vigilant, critical, and unapologetically raw. The future is uncertain, and that’s what makes it so damn exciting. So crank up the volume, let the beats wash over you, and join the revolution—a revolution where every split stem is a reminder that, in the end, music is more than just sound. It’s a living testament to our collective soul, a dance between chaos and order that will continue to evolve, inspire, and defy expectations.